Technological utopianism is the belief that technical progress always benefits in the long run and so will automatically lead to the end of sickness, hunger, poverty and even death itself. Yet as critics point out, such techno-idealism is not fact-based:
“The unfulfilled promise of past technologies rarely bothers the most fervent advocates of the cutting edge, who believe that their favorite new tool is genuinely different from all others that came before. And because popular belief in the world-saving power of technology is often based on myth rather than carefully collected data or rigorous evaluation, it is easy to see why technological utopianism is so ubiquitous: myths, unlike scientific theories, are immune to evidence.” Evgeny Morozov
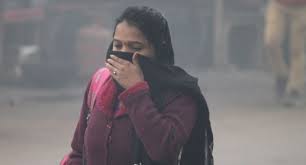
The techno-realist approach taken here suggests that technology harms as well as helps. In the area of health, Richard Lear points out that while traditional heart and cancer rates are steady, there has been a rapid rise in the rates of diseases like autism (2094%), Alzheimer’s (299%), diabetes (305%), sleep apnea (430%), celiac disease (1111%), ADHD (819%), asthma (142%), depression (280%), bipolar youth (10833%), osteoarthritis (449%), lupus (787%), inflammatory bowel (120%), chronic fatigue (11027%), fibromyalgia (7727%) and multiple sclerosis (117%) since 1990. This unprecedented explosion of autoimmune, nerve, metabolic and inflammatory diseases in one generation seems related to our use of technology. Doctors argue that sitting at a computer is the new smoking. Others blame technologizing the planet for heat waves, city smog, sea levels, forest fires, hurricanes and droughts. Experts point out that technology is unlikely to fix these problems. So maybe technology is killing us?
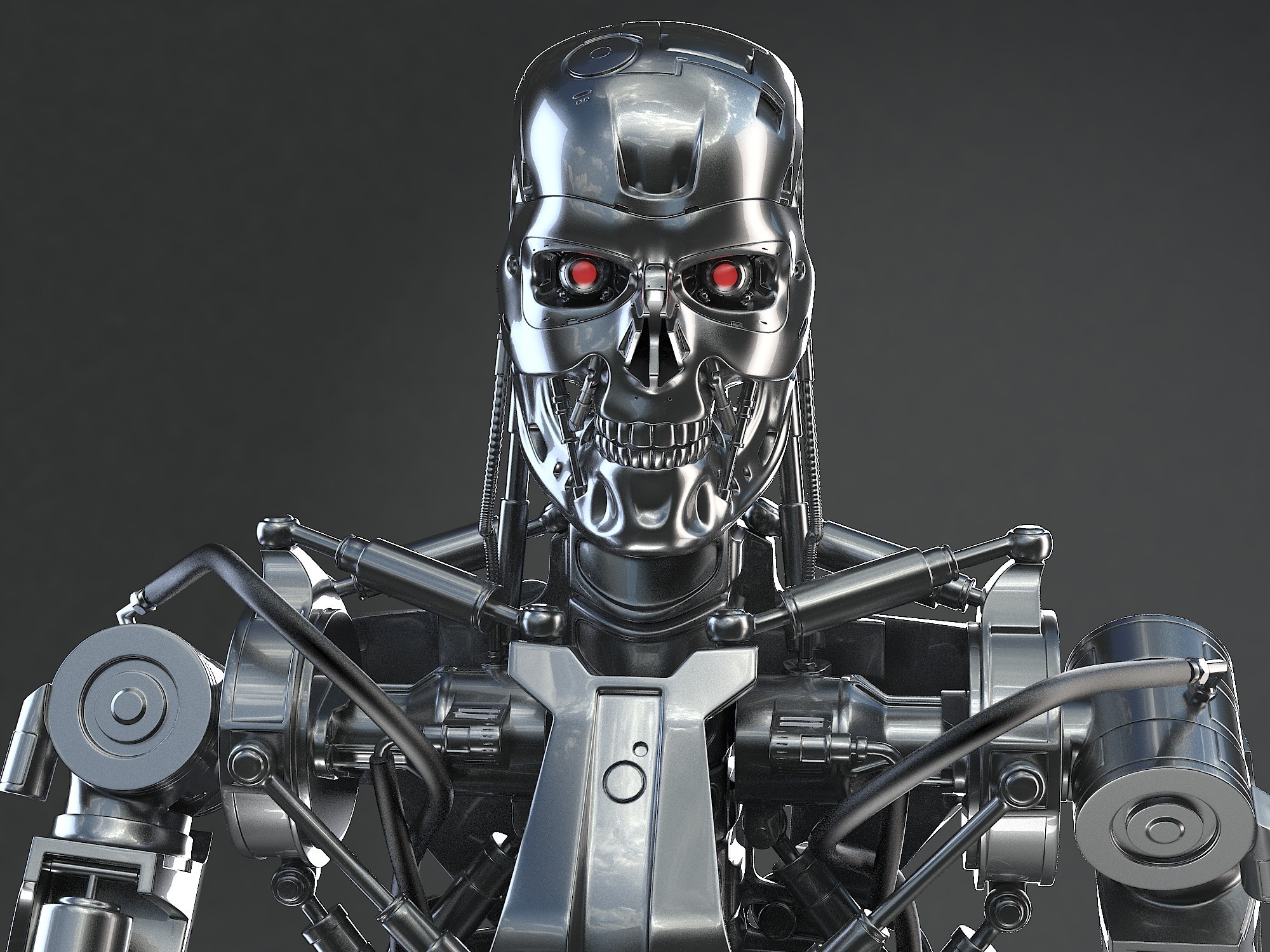
The myth of the robot. Technological utopianism goes a step further, claiming that computers will soon surpass humans by Moore’s law, that computer power doubles every eighteen months. In Figure 7.3 computers processed as an insect in 2000, as a mouse in 2010 and will exceed the human brain in 2025. In this view, computers are an unstoppable evolutionary juggernaut, but fast-forward to 2018 when while engineers have made tiny robo-flies, computing still struggles to do what flies do with a neuron sliver:
“The amount of computer processing power needed for a robot to sense a gust of wind, using tiny hair-like metal probes embedded on its wings, adjust its flight accordingly, and plan its path as it attempts to land on a swaying flower would require it to carry a desktop-size computer on its back.” Cornell University
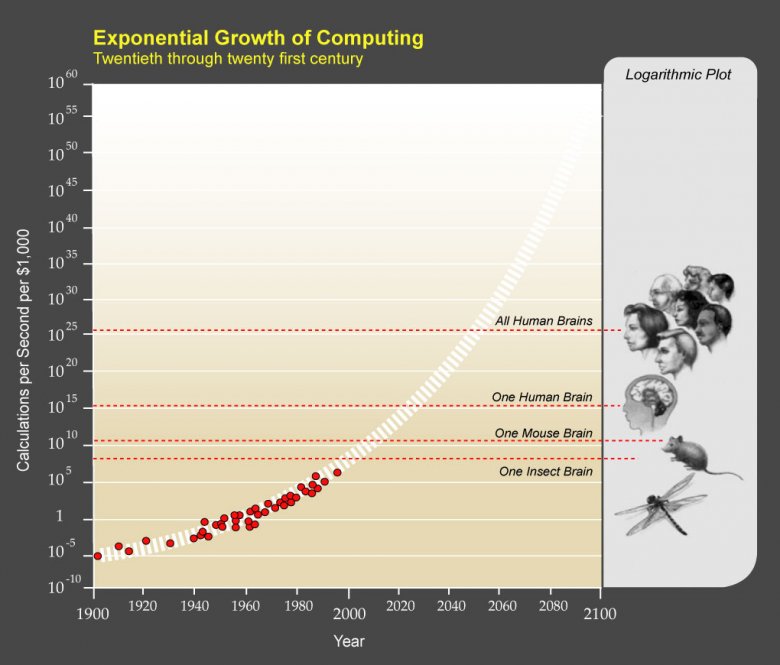
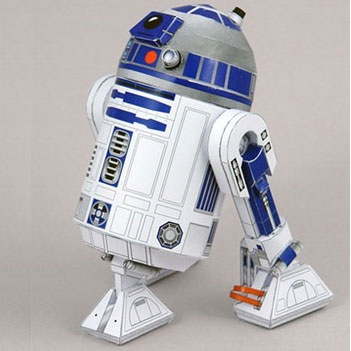
Cornell engineers conclude they will need new “event-based” algorithms running on a non-traditional chip architecture to mimic neural activity. Yet the myth of the robot remains popular science-fiction; e.g. Rosie in The Jetsons, C-3PO in Star Wars and Data in Star Trek are robots that read, talk, walk, converse, think and feel. We do these things easily, so how hard could it be? In movies, we see robots that learn (Short Circuit), reproduce (Stargate’s replicators), think (The Hitchhiker’s Guide’s Marvin), become self-aware (I, Robot), rebel (Westworld) and then replace us (The Terminator, The Matrix). What is not apparent to most is that the myth of the robot is the centuries old myth of the machine re-incarnated in a modern context.
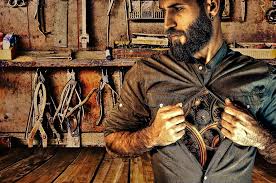
The myth of the machine. By the 19th century, many felt that the universe was just a clockwork machine where as Laplace said:
“We may regard the present state of the universe as the effect of its past and the cause of its future. An intellect which at a certain moment would know all forces that set nature in motion, and all positions of all items of which nature is composed, if this intellect were also vast enough to submit these data to analysis, it would embrace in a single formula the movements of the greatest bodies of the universe and those of the tiniest atom; for such an intellect nothing would be uncertain and the future just like the past would be present before its eyes.” Laplace, A Philosophical Essay on Probabilities
Laplace was saying, in a complex way, that if I knew what every atom in your brain was doing, I could predict your thoughts and acts. In a clockwork universe the future is set, including who you fall in love with, what your job is and when you die. It’s all just physics but a century later physics found that reality isn’t like that at all. Quantum theory killed the clockwork universe because Heisenberg’s uncertainty principle doesn’t let us measure what particles are doing now fully. Quantum events multiply, so to simulate a hundred electrons would take a computer bigger than the earth, see here, so to simulate even a big atom like Uranium would require extra planets! This changes the estimate for when computers will simulate the brain from decades to millions of years. Physics does not support classical computing claims because quantum particles interact exponentially, a phenomenon linguistics calls productivity.
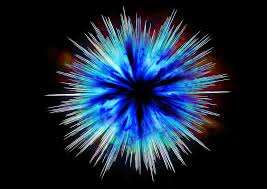
Reality is productive. The myth of the machine implies that people are machines, so in psychology Behaviorism argued that people were fully explained by their input and output behavior. Chomsky’s answer was to note that the productivity of language is such that five year olds can speak more different sentences than they could learn in a lifetime at one sentence per second (Chomsky, 2006), so they couldn’t be just learning behaviors. The myth of the robot fails when possible sentences explode, just as the myth of the machine fails when possible events explode in quantum calculations. A physical explosion is when one atom chemically interacts with others that do the same in rapid escalation and likewise information explodes when choices interact to cause other choices. The same occurs in other fields like genetics and sociology, that when many elements interact the choices soon go beyond classical calculations. That calculations fail when information explodes is illustrated by the 99% barrier.
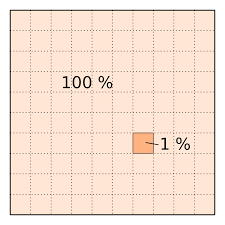
The 99% barrier. The 99% barrier has bugged AI from the beginning, e.g. 99% accurate computer voice translation is one error per minute, which is unacceptable in conversation. Likewise 99% accuracy for robot cars is an accident a day, again unacceptable! Since a good driver’s mean time between accidents (MTBA) is about 20 years, real life requires better. Yet getting that last percentage is inordinately hard, as 100% accuracy is impossible in an indeterminate world. Hence robot successes are either “almost there” like the robofly or involve unreal scenarios like chess or Go. Getting a driverless car to work in an ideal setting is not enough, as the CEO of a Boston-based self-driving car company says:
“Technology developers are coming to appreciate that the last 1 percent is harder than the first 99 percent. Compared to last 1 percent, the first 99 percent is a walk in the park.” Karl Iagnemma
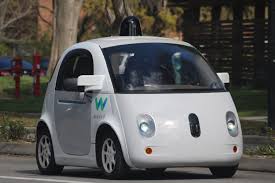
The reality is that robot car fatal accidents are a matter of when not if. And it has already happened, as when a driverless Uber car killed a jaywalking pedestrian after only 2 million miles versus the US 1.18 deaths per 100 million miles average for people. And how often do human minders have to intervene? The current Waymo rate of every five thousand miles means minders have to pay attention all the time, so the passenger might as well be driving. Add in bad weather, system failures and unusual events and it isn’t hard to see why Uber recently halted self-driving car tests. Realistically, one can expect robo-cars to debut as a form of 25mph public transport in restricted no-car areas, not on main roads. The last 1% will not give up easily!
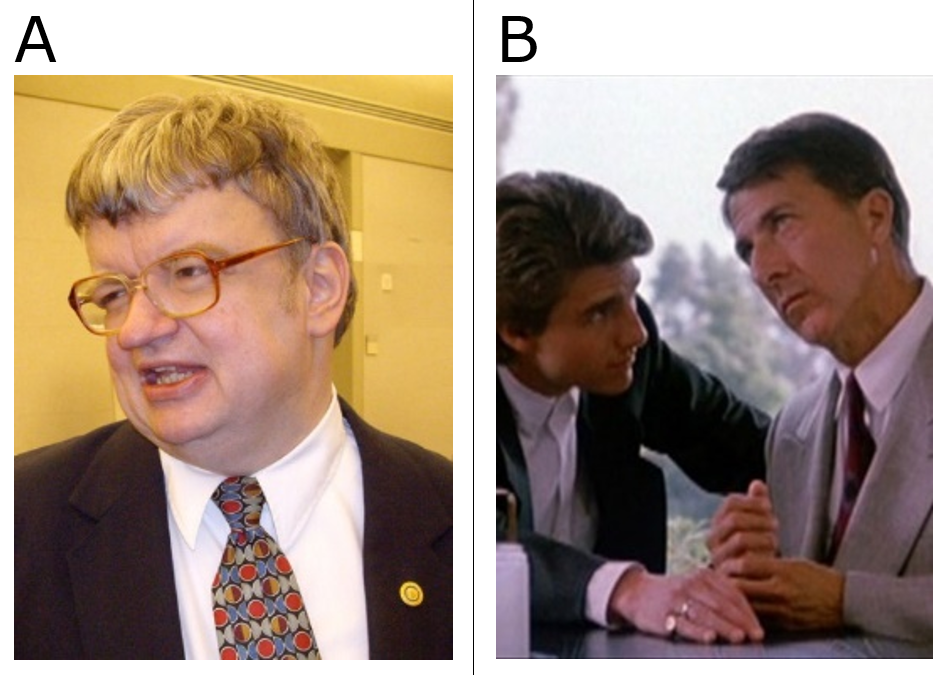
Calculating alone can’t solve real life tasks. The brain did not cross the 99% performance barrier by calculating more because it cannot be done. Simple processing power depends on neuron numbers but advanced processing does not. Our brain, as it evolved, found this out by trial and error. In savant syndrome, people who can calculate 20 digit prime numbers in their head need full time care to live in society; e.g. Kim Peek, who inspired the movie Rain Man, could recall every word on every page of over 9,000 books, including all Shakespeare and the Bible, but had to be looked after by his father to live in society (Figure 7.5). He was a calculating genius but in human terms was neurologically disabled, as the higher parts of his brain didn’t develop. Savant brains are brains without the higher sub-systems that allow abstract thought. They calculate better because the brain tried simple processing power in the past and moved on. Computers are electronic savants, calculation wizards that need minders to work in a real world.
Beyond calculations. Von Neumann designed the first computer and almost every computer today still follows his architecture. He made these simplifying assumptions to ensure success:
- Control: Centralized. All processing is directed from a central processing unit (CPU).
- Input: Sequential. Input channels are mainly processed in sequence.
- Output: Exclusive. Output resources are locked for single use.
- Storage: Location based. Information is accessed by memory address.
- Initiation: Input driven. Processing is initiated by input.
- Self-processing: Minimal. Minimize self referential processing to avoid paradoxes and infinities.
How did the brain manage “incalculable” tasks given that it is an information processor? Its trillion (1012) neurons are biological on/off devices powered by electricity, in principle no different from transistors. The brain crossed the 99% performance barrier by taking the design risks Von Neumann avoided, namely:
- Control: Decentralized. Processing sub-systems work on a first come first served basis.
- Input: Massively parallel. Input channels are massively parallel, e.g. the optic nerve.
- Output: Overlaid. Primitive and advanced sub-systems overlap in output control.
- Storage: Connection based. Information is stored by connection patterns.
- Initiation: Process driven. Processing initiates, to hypothesize and predict.
- Self-processing: Maximal. Processing of processing gives us a “self” to allow social activity.
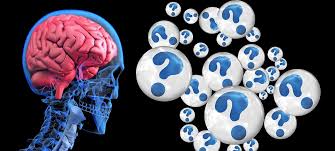
Super-computers can maximize processing by running NVidia graphic cards in parallel but the brain went beyond that. Its biological imperative was to deal with uncertainty not to calculate it. Technical utopianism assumes that all processing is simple processing but by repeated processing upon processing the brain came to construct a self, others and a community, the same constructs that human and computer savants struggle with. Instead of an inferior biological computer, the brain is a different type of processor, so today’s super-computers aren’t even in the same processing league as the brain. Computers calculate better than us as cars travel faster and cranes lift more, but the brain evolved evolved to handle reality not to calculate it. If today’s computers excel at the sort of calculating the brain outgrew millions of years ago, how are they the future? AI will never surpass HI (Human Intelligence) because it is not even going in the same direction.