Causality. Years ago, scientists reported that peanuts caused cancer so my dad immediately gave them up for the rest of his life, yet nuts are now seen as healthy. How can a science based on facts get things like that wrong? The answer is by seeing causality that is not so. If facts are the data we measure, then causality isn’t a fact because we infer rather than see it directly, e.g. Newton saw gravity as a force from the earth pulling the apple down, but no-one ever saw gravity so it wasn’t a “fact”. This is why Einstein could later show that masses don’t emit forces but just change the space and time around them. Causes are not facts but inferred from facts, so are easy to get wrong. A common error is to infer causation from correlation.
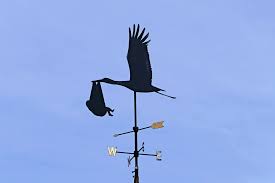
Correlation is not causation. Folklore shows pictures of storks delivering babies but a study has shown that the correlation between the number of storks and the number of babies delivered is a strong 0.62 and very significant at p < 0.01. So do storks deliver babies? Equally there is a correlation between number of ice-creams consumed and number of drownings, so does eating ice-cream cause drownings? In both cases, the correlation arises not because one causes another but because a third thing causes both. Storks correlate with babies because the weather makes both go up and down together. Likewise ice cream correlates with drownings because in the summer swimming and eating ice cream go up and in the winter both go down, giving a spurious correlation. To understand this, explain why that the shoe size of children correlates with mathematics ability doesn’t mean you should buy your child bigger shoes!
Correlation logic. In logic, that one thing causes another can be written X→Y and that the two correlate can be written X~Y. Correlation then does not mean causation because that X~Y could mean that:
- X causes Y: X→Y, or
- Y causes X: Y→X, or
- A third variable Z causes both: Z→X and Z→Y.
So in the stork case, X=Number of storks, Y=Number of babies and Z= Weather. Another example is the correlation between the number of violent movies watched by teenagers and their tendency to violence. One can infer that watching violent movies causes violence but it is equally likely that violent teenagers prefer violent movies, i.e. that the causation works the other way. If you think science is immune to such errors, think again. Studies showing that women on hormone replacement therapy had a lower coronary heart disease led doctors to conclude it was protective until controlled studies found no such effect. They got the causality backwards – it wasn’t hormone therapy making the women healthier but that women from higher socio-economic groups with better-than-average diet and exercise regimens could afford the therapy. The point is important because research in areas such as health and marketing are full of causal inferences made from correlational data.
Science and causation. To the statement that correlation is not causation it must be added that no degree of significance ever establishes causation. Significance only tells that the result is not random, not what causes what. To show causality, science must not just look at data but also manipulate it. The experimental method holds everything else constant then manipulates the proposed cause, so it is the research design that establishes causality, not the statistics. Rather than just observing a cause, one changes it to see the effect, i.e. by acting not just looking. If this is impossible, one needs a known mechanism, e.g. research linking smoking to cancer is correlational as it is unacceptable to raise one group of babies with cigarettes and another without! Causality is inferred because smoke is known to be carcinogenic.
Progressing to causality. Research methods can be seen as progress to causality:
- Discovery. Methods like grounded theory discover important constructs.
- Description. Qualitative methods describe constructs better.
- Correlation. Quantitative observations find construct correlations.
- Causality. Experiments manipulate constructs to show causality.
Thus the different methods of science are complementary not in competition.
Error types. The two types of error are false positives and false negatives. The worst result in research is not “negative” data that contradicts a theory but data that shows nothing at all, or nil results. Nil results are random data that has no meaning or value. Perhaps the literature review badly defined constructs, or confounded two effects that cancelled out, or measurement error “noise” drowned out an effect. And even if results are obtained they may be in error. Research error comes in two types:
- Type I error. Type I error is a false positive result. It is an error of commission.
- Type II error. Type II error is a false negative result. It is an error of omission.
False positives occur when research doesn’t have enough rigor. Research rigor includes reliable and valid measures, a bias-free method and correct analysis including testing any assumptions. It ensure that any result found is unlikely to be random or due to causes other than those proposed. Research must be rigorous enough to avoid false results.
False negatives occur when research does not have enough sensitivity. Improve research sensitivity by methods that enhance responses, e. g. motivate subjects to be honest, reduce subject error, e.g. subject training, or more sensitive statistical tests. Research must be sensitive enough to register results.
Reducing one error type tends to increase the other. Research is often a trade-off between rigor and sensitivity, as too much rigor results in not finding real effects and too much sensitivity results in finding false effects. It is like in life, where taking every precaution to avoid danger gives missed opportunities, and taking every opportunity gives more danger. In general, reducing false positives to zero increases false negatives to 100% and vice-versa.